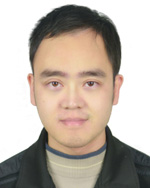
Le Chang
Institute of Neuroscience
email: lechang@ion.ac.cn
address: Room 430, ION Building, 320 Yue Yang Road
Research Areas
high-level vision; neural mechanism of object recognition; system neuroscience; deep learning
Education
2006-9~2014-1, Institute of Biophysics, Chinese Academy of Sciences, PhD degree
2002-9~2006-7, Nankai University, Bachelor's degree
Experience
Work Experience
2018-1~present, Institute of Neuroscience, Chinese Academy of Sciences, Investigator
2013-9~2017-12, California Institute of Technology, Postdoctoral scholar
Publications
Papers
1.Le Chang, Pinglei Bao and Doris Tsao. (2017). The representation of colored objects in macaque color patches. Nature Communications, 8: 2064.
2.Le Chang* and Doris Tsao*. (2017). The code for facial identity in the primate brain. Cell, 169 (6): 1013-1028. (*co-corresponding author)
3.Sebastian Moeller, Trinity Crapse, Le Chang and Doris Y Tsao. (2017). The effect of face patch microstimulation on perception of faces and objects. Nature Neuroscience, 20, 743–752.
4.Le Chang* and Shigang He*. (2014). Light adaptation increases response latency of alpha ganglion cells via a threshold-like nonlinearity. Neuroscience, 256:101-116. (*co- corresponding author)
5.Tom Baden#, Timm Schubert#, Le Chang, Tao Wei, Mariana Zaichuk, Bernd Wissinger and Thomas Euler. (2013). A tale of two retinal domains: near optimal sampling of achromatic contrasts in natural scenes through asymmetric photoreceptor distribution. Neuron, 80 (5): 1206-1217.
6.Le Chang, Tobias Breuninger and Thomas Euler. (2013). Chromatic coding from cone-type unselective circuits in mouse retina. Neuron, 77(3): 559-571.
7.Tao Wei, Timm Schubert, François Paquet-Durand, Naoyuki Tanimoto, Le Chang, Katja Koeppen, Thomas Ott, Oliver Griesbeck, Mathias W. Seeliger, Thomas Euler and Bernd Wissinger. (2011). Light-Driven Calcium Signals in Mouse Cone Photoreceptors. J. Neuroscience, 32(20): 6981-6994.
Research Interests
One pivotal function of the brain is to identify
objects in the surroundings based on sensory inputs. Primates heavily
rely on their visual system to extract object-related information.
Photoreceptors in the retina pick up light reflected by the environment,
and the primate brain is able to identify the objects causing the
reflection. This is a remarkable ability, given that the reflected light
of the same object could differ tremendously under different
conditions, such as the lighting condition, the position and orientation
of the object, presence of occlusion etc., however, we are able to
recognize the object regardless of these variations. Inferotemporal (IT)
cortex is believed to be the brain region responsible for object
recognition: patients with lesion to this brain region showed deficits
in their ability to recognize objects; furthermore, neurons in IT are
selective to objects irrespective of accidental changes, such as
position, size and orientation. By combining functional MRI,
electrophysiology and computational modeling, our lab aims to understand
how different types of objects, such as faces, bodies and fruits, are
represented in IT cortex of the monkey brain. In particular, we are
interested in the following topics:
1. Understanding the
neuronal computation of facial identity in IT. Faces are very important
objects for the animal, and previous studies discovered a subnetwork of
IT, the face patch system, specialized for face processing. Cells in
face patches were shown to code facial identity independent of
accidental changes, however the detailed computation implemented by face
patch neurons are still not clear. Our goal is to construct a
computational model as close as possible to simulate the function of
face patch neurons. So far, most of our knowledge on these cells has
been obtained by presenting simple un-occluded facial images to the
monkey. To better constrain the model, we will expand the set of images
to include more variations, such as occlusion, clustering and
scrambling—such variations may or may not change the responses of face
cells, and our model should simulate real cells in all cases. After we
obtain such a model, we could perform in-silico analysis to understand
how facial identity is computed in detail.
2. Understanding the representation of objects in IT. Faces are very special objects: they generally have similar shapes but we are able to identify subtle changes in faces. The space of all objects is much more complex and heterogeneous than face space, and the representation of general objects in IT remains a big mystery. The key question we want to address is: what are the factors governing the organization of objects in the responses of IT cells. For example, two very general factors have been proposed: the semantic meaning of the object (such as animate/inanimate objects) and the shape of the object (such as roundish/square objects)—furthermore, both factors could be further decomposed into more specific features. To explore this question, we will first construct a computational model of IT cells similar to face cells, and then test different possible factors in the model. The likely candidates screened by the model will be further tested on cells in the monkey brain.
Conferences
(2)Recordings from macaque face patches reveal a mechanism for view-invariant computation, Annual Sloan-Swartz Meeting, 2015-08-03
(3)Representation of a parameterized realistic face space across different face patches, Annual meeting of Society for Neuroscience, 2014-11-17